Research Background
The idea behind bio-inspired electronics is trying to understand and reproduce nervous biological systems through integrated circuits with a kind of bottom-up approach, starting with simple neurons or low-level sensory systems up to high-level perceptive cognitive systems made up of neuronal ensembles and multisensory integration. Key features are event-driven data flow, time-encoded information, mixed-signal integration, and low power consumption.
The applications include edge computing of internet-of-things (IoTs), robotics, unmanned aerial vehicle (UAV), satellites, and underwater unmanned systems, where common “traditional” sensory, information processing and communication, and control parts could be shifted to this new paradigm, where numeric data are progressively replaced by asynchronous mixed-signal events, thereby requiring a significant change in the design and development approaches.
To implement the bio-inspired electronic system, the technical approaches are mainly composed of “Fundamental theories of bio-inspired sensory and computing”, “bio-inspired sensors”, “bio-inspired computing processors” and “nanoscale mixed-signal integration”.
Fundamental Theories of Bio-inspired Sensing and Computing
The new paradigm of bio-inspired computers is based on the fundamental algorithms which are learned from biological systems. New theories, new materials, and new devices are investigated to explore new applications. In addition, fundamentals of bio-inspired mathematics including simulated evolutionary computing theories, artificial neural network theories, fuzzy logic, and fuzzy inference should be deeply studied. Then, novel bio-inspired algorithms such as ant colony algorithm, particle swarm optimization algorithm, artificial bee colony algorithm, differential evolution algorithm, cultural algorithm, memetic algorithm, and artificial immune algorithm can be improved to construct new computing architectures. The objective of these research topics is to make the bio-inspired computers “Perceive” and “Thinking” as intelligent creatures.
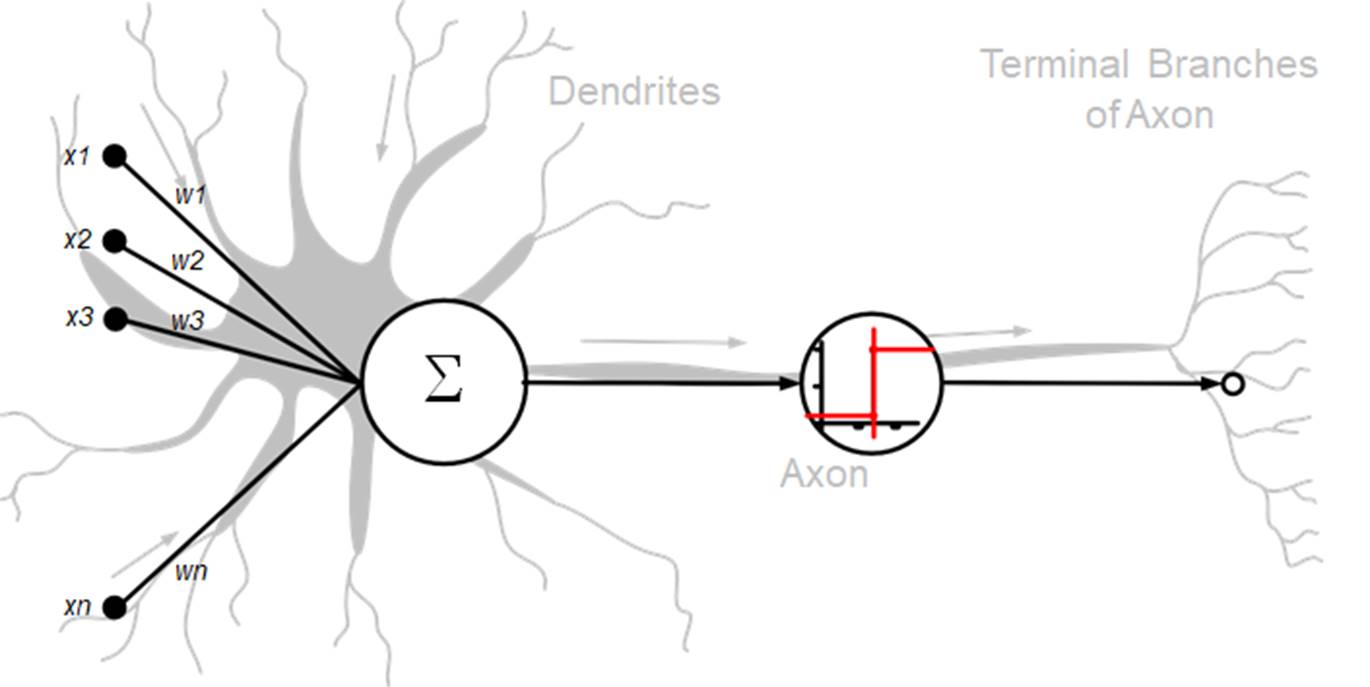
(Source: “Neuronal Dynamics”, 2013)
Bionic Electronic Sensors
Nature is an excellent "engineer". In the long process of evolution, animals, plants and microorganisms have obtained their own perception systems. Taking human beings as an example, the five senses and skin convert external stimuli into nerve pulse electrical signals through vision, hearing, smell, taste and touch, so that we can perceive the world. The development of bionic electronic sensors simulates the human perception system, detects various stimuli from the world, and gradually surpasses the human sensory level in sensitivity, selectivity and accuracy, so as to help people understand the unknown world and facilitate our daily life. Since 80% of the information is obtained by eyes, bionic vision sensors (not only a CMOS image sensor) are the most important sensors for future AI systems. A novel image sensor implemented by ultra-small pixels, large-scale arrays, wide-spectrum detection and near-sensor/in-sensor computing circuits is required. In addition, the bionic auditory sensor is the basis of dialogue with an intelligent system. New bionic auditory sensors are realized with high-precision MEMS sensors with low-power in-sensor computing electronics. Moreover, bionic olfactory and taste sensors will be developed to extend the application of AI machines. Novel “Electronic Nose” and “Electronic Tongue” are required to let the machine “smell” and “taste”. Bionic electronic skin is also important to sense and recognize pressure, temperature and humidity.
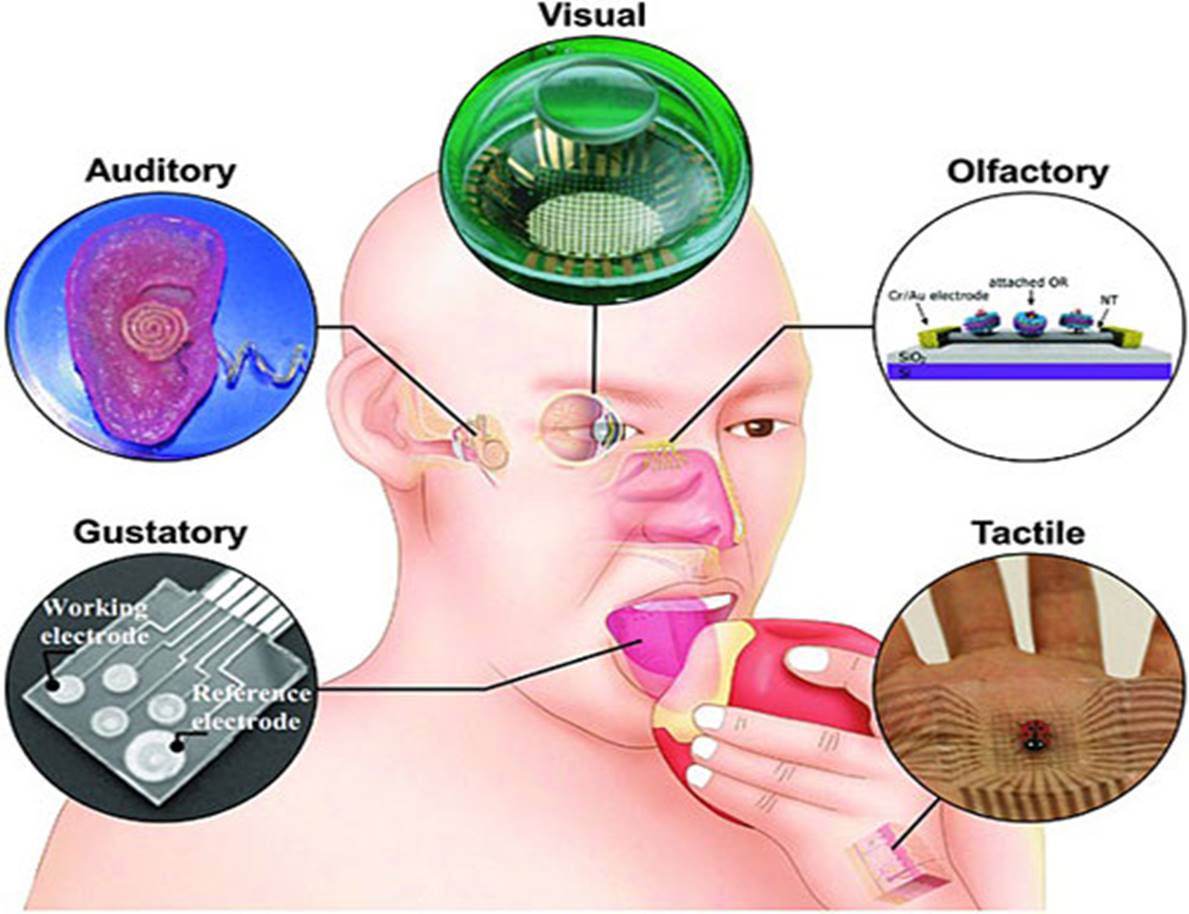
(Source: Adv. Mater.)
The current task in this direction is to design a pixel readout ASIC with in-memory computing for pixel detectors. In the imaging fields of visible light, ultraviolet light, infrared light and terahertz, pixel readout ASIC is one of the key devices of hybrid focal plane pixel detectors. Using pixel readout ASIC, the charge signals of the two-dimensional array sensor can be read out and digitized via point-to-point analog front-ends. This technology can also be extended to the signal processing of olfactory array sensors, gustatory array sensors and pressure sensors. A digital pixel readout circuit is a newly developed structure. In this structure, the digital data which are stored in a local memory within the pixel can be generally read out from the sensor by "Parallel-In-Serial-Out (PISO)" approach, and then processed by digital signal processing. Such a structure has several issues in signal processing, such as "data transmission bottleneck", "memory wall" and "power consumption wall". In order to solve these problems, the information processing directly at the sensor end is proposed in recent years. One idea is to integrate digital pixel readout circuits and memory computing circuits to realize an architecture of sensing, storage and computing. Therefore, it is necessary to explore the intelligent sensor architecture combining pixel sensor readout, in-memory computing and deep learning accelerator together.
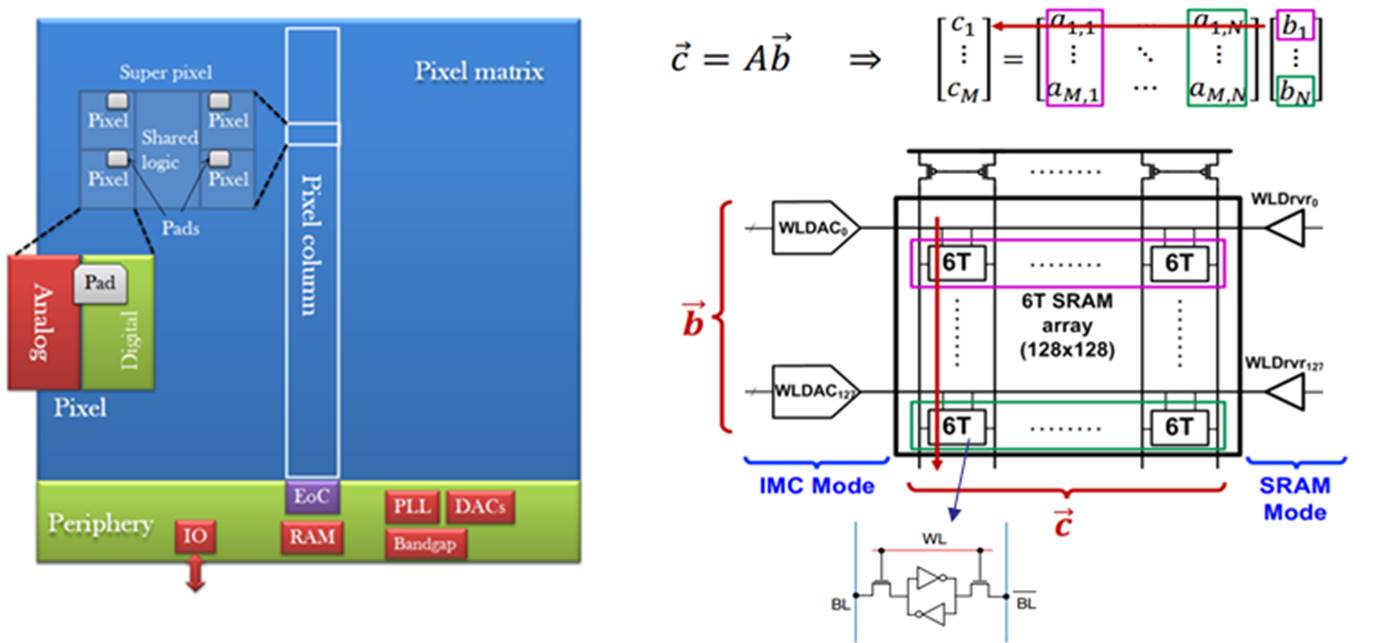
(Source: J. Zhang,2017)
Bionic Computing System-on-Chip (BioSoC)
A bionic computing system-on-chip (BioSOC) is an artificial intelligence processor that adds an independent processing unit dedicated to neural network computing on the basis of the original processor chip. It is a hardware mode of algorithm capabilities such as speech and picture recognition, classification, inference, motion control and acceleration. The implementation of such SoC is based on a heterogeneous computer architecture and the mixed-signal integration. Take the bionic chip of mobile phones from Apple Company as an example. The specific description of the bionic chip is: (a) Apple bionic chip actually integrates an independent processing unit with AI computing capability on the original A-Series SOC. It is the same thing as the NPU that often appears in other mobile phone chips, but its name is different. (b)The core part of Apple bionic chip includes CPU, GPU and NPU. The CPU is responsible for general computing, which can complete various complex tasks and has very strong adaptability; GPU is responsible for processing graphics tasks, including rendering of images and models; NPU is responsible for Artificial Intelligence Computing, including speech and image recognition, face recognition, etc.(c)Independent NPU chip can realize the advantages of small volume, low power consumption, high reliability and strong confidentiality. Compared with relying solely on CPU or GPU for some algorithm processing, NPUs which sever domain-specific computing is more in line with the current trend. Since CPUs and GPUs are mature devices, domain-specific NPU is the focus of research in the next few years.
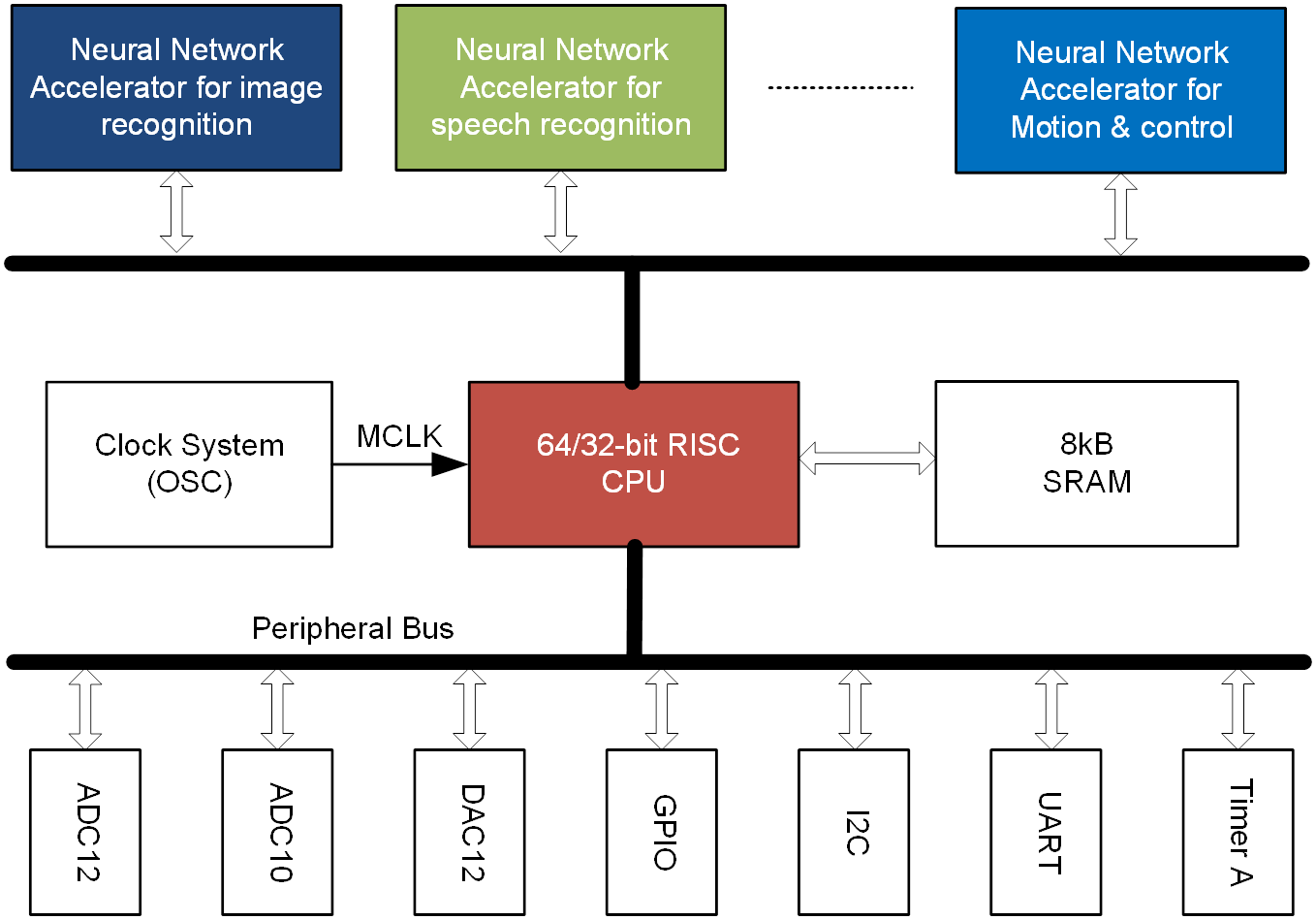
(Source: W. Gao, 2021)
Nanoscale Mixed-Signal Integration
Nanoscale CMOS technology is a common solution to implement a bio-inspired sensing and computing system on a chip. In the past, neuromorphic computing chips were usually realized by very large-scale digital circuits. However, during the research and development (R&D) of the second generation of deep learning processors, it is found that AI chips realized by digital circuits have the problems of "memory wall" and "power wall" even if they adopt the most advanced CMOS technology, which limits the energy-efficiency ratio of AI Computing. The AI computing core realized by the existing digital circuit can reach the energy efficiency ratio of 1 TOPS/W in 2020. To further improve this value, emerging technologies need to be adopted. Recently, IBM released a solution on the company's official website. They proposed to improve the energy efficiency ratio of AI chips by 1000 times through three steps in the development roadmap in the next decade. In the first step, the digital AI core with approximate calculation will be used to improve the energy efficiency ratio to 10 TOPS/W. In the second step, the AI computing core with analog circuits will be used to improve the energy efficiency ratio by another 10 times. In the third step, the AI computing core with analog circuits of emerging materials will be used to improve the energy efficiency ratio to 1000 TOPS/W. Therefore, the implementation of next-generation AI chips based on mixed-signal integrated circuits is the hotspot and the trend of AI chips in the future.
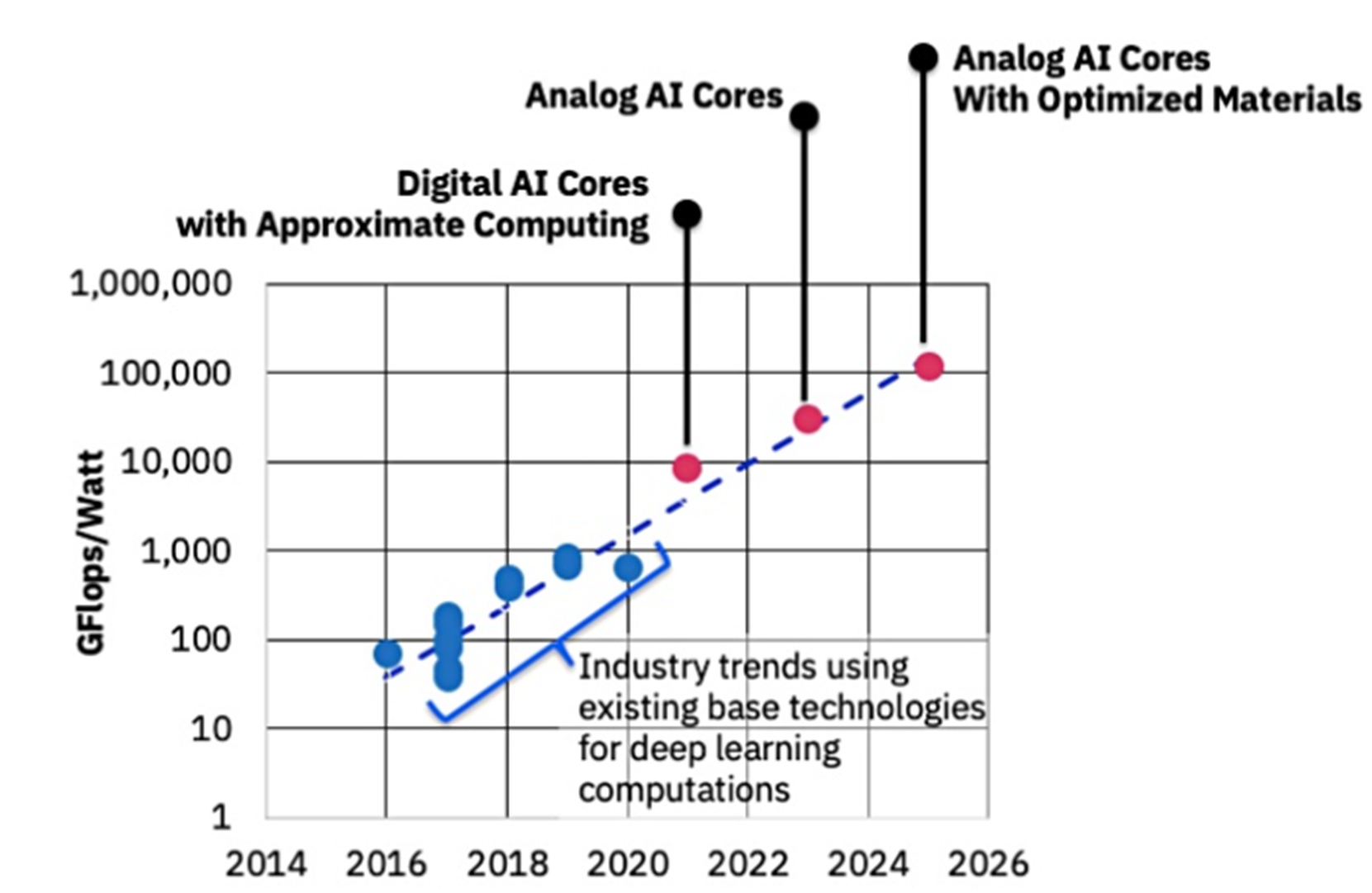
(Source: IBM website)